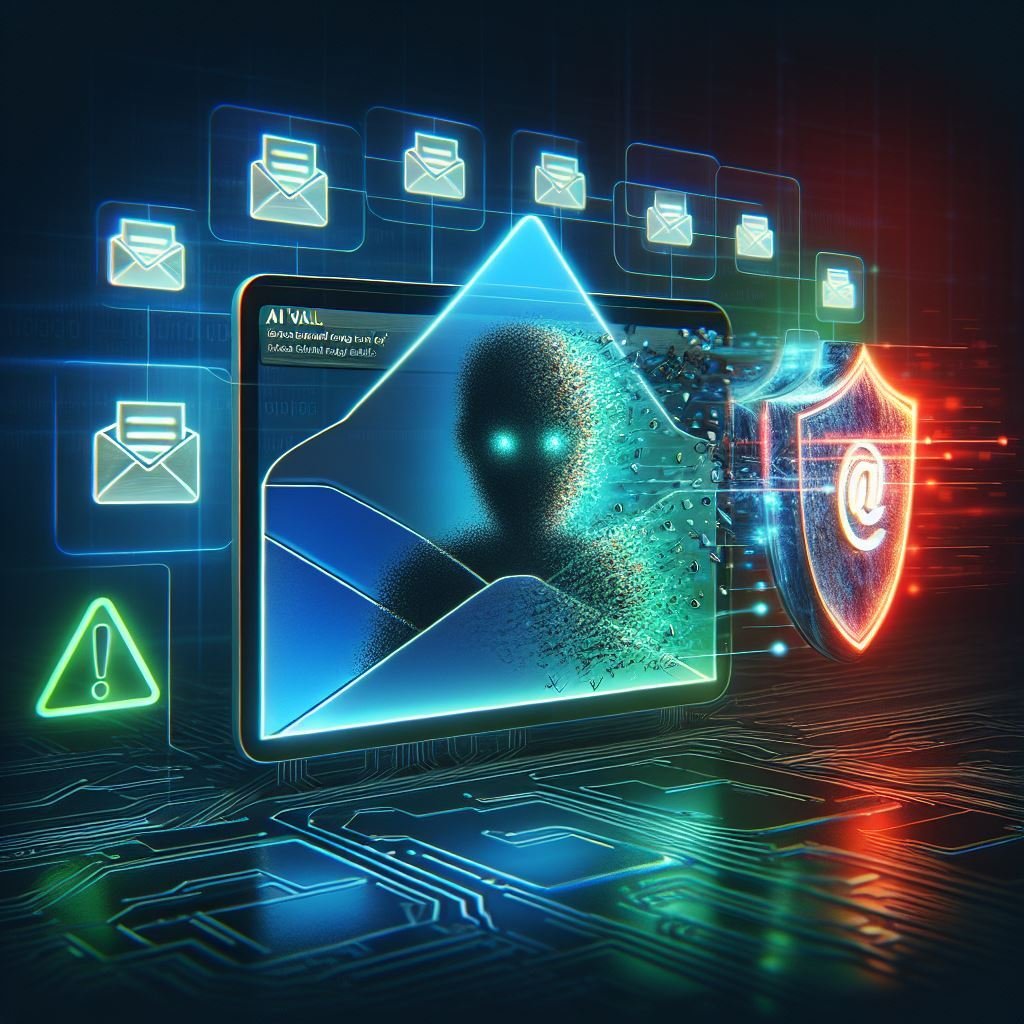
Are Traditional Data Security Approaches and Tools Insanity?

Albert Einstein famously said, "Insanity is doing the same thing over and over again and expecting different results." This quote resonates deeply when we consider the idea of data security, where companies persistently employ traditional approaches and tools, only to encounter the same frustrating outcomes. Despite the ever-evolving landscape of cyber threats and the fast adoption of artificial intelligence (AI), the process of discovering everything, classifying it, defining numerous rules, and attempting to enforce them has proven to be ineffective time and time again.
The Traditional Approach: A Vicious Cycle
For years, organizations have relied on an established data security strategy that involves several key steps and is deployed in a rinse-and-repeat fashion regardless of the type of company or industry. The names of the tools and processes may vary, but the overall structure and what those tools and processes accomplish does not.
- Discovery: Identifying all data assets within the organization.
- Classification: Categorizing data based on sensitivity and importance.
- Rule Definition: Establishing a comprehensive set of security policies and rules.
- Enforcement: Implementing these rules across the organization to protect data.
While this approach seems logical on paper, it often falls short in practice. Let’s dive into the why.
The Challenges of Traditional Data Security
The process of classifying data is incredibly complex and resource intensive. By the time data classification is complete, it is often outdated and not granular enough to be relevant to the outcomes desired by the business. Organizations often struggle to keep up with the sheer volume of data, leading to incomplete or outdated classifications. This complexity and overhead can be overwhelming, making it difficult for companies to maintain accurate and up-to-date data inventories, limiting the ability to enforce data rules.
Defining a multitude of security rules can create a rigid framework that becomes difficult to adapt to new threats. Cybercriminals are constantly evolving their tactics, and static rules can quickly become obsolete. This rigidity in rule definition means that organizations may find themselves ill-equipped to handle emerging threats, leaving their data vulnerable.
Enforcing security policies consistently across an organization is a monumental task. Human error, lack of awareness, and technological limitations can all contribute to enforcement gaps, leaving data exposed. These enforcement gaps highlight the challenges organizations face in maintaining a robust security posture.
Traditional approaches to data security are often reactive, addressing threats after they have already occurred. This reactive stance leaves organizations playing catch-up, rather than proactively preventing breaches. As a result, companies may find themselves constantly responding to incidents instead of focusing on preventing them in the first place.
And by far, the biggest challenge with traditional data security tools is that they were built primarily to manage data at rest, including databases and cloud storage. For content that is generated on the fly, such as emails and chats, traditional tools will struggle even more as manual data classification is just not practical.
Stop the Insanity
Given these challenges, it's clear that a new approach to data security is needed—one that moves beyond the traditional methods that have repeatedly failed to deliver the desired results, i.e. the insanity. Here are some key elements of a more effective data security strategy designed for the world of AI:
- Adaptive Security: Instead of relying on static rules that quickly become outdated, organizations must adopt adaptive security measures that can learn and evolve in response to emerging threats. This includes leveraging machine learning and artificial intelligence to detect and respond to anomalies in real-time.
- Zero Trust Model: The Zero Trust security model operates on the principle of "never trust, always verify." This approach assumes that threats can come from both inside and outside the organization and requires continuous verification of user identities and access privileges.
- Data-Centric Security: Rather than focusing solely on perimeter defenses, organizations must implement data-centric security measures that protect data at the source. This includes encryption, tokenization, and robust access controls.
- Continuous Monitoring and Response: Proactive security requires continuous monitoring of data and systems to detect and respond to threats in real-time. This involves using advanced analytics and threat intelligence to stay ahead of cybercriminals.
- User Education and Awareness: Human error is a significant factor in data breaches. Investing in user education and awareness programs can help employees recognize and respond to potential threats, reducing the risk of accidental data exposure.
The AI Challenge: A New Frontier in Data Security
As AI becomes increasingly integrated into everyday business operations, it introduces new complexities and challenges for data security. Traditional data security tools and processes are insufficient to address the unique risks posed by AI. Here are some of the key challenges:
- Increased Complexity: AI systems often require vast amounts of data for training and operation. This influx of data can overwhelm traditional security tools, making it difficult to maintain comprehensive oversight and control.
- AI-Driven Threats: AI can be used by cybercriminals to launch more sophisticated attacks, such as deepfakes, automated phishing, and AI-powered malware. These threats are harder to detect and mitigate using traditional security measures.
- New Attack Vectors: AI introduces new attack surfaces, such as machine learning models and data pipelines. Traditional security measures may not be equipped to protect these components, leaving them vulnerable to exploitation.
- Data Integrity Risks: AI systems rely on vast amounts of data to function effectively. Ensuring the integrity and security of this data is crucial, as compromised data can lead to incorrect AI outputs and decisions.
- Privacy Concerns: AI applications often involve processing large volumes of personal data, raising significant privacy concerns. Traditional data security tools may not be equipped to handle the privacy implications of AI.
- Regulatory Challenges: As AI technology evolves, so do the regulatory requirements surrounding data security. Organizations may find it challenging to keep up with these changes and ensure compliance using their existing security frameworks.
- Resource Strain: The need for continuous monitoring and updating of AI systems can strain existing security resources. Traditional tools may not be capable of providing the real-time analysis and response required to protect AI environments.
Embracing AI-Enhanced Security
To effectively secure AI-driven environments, organizations need to adopt new strategies and tools that leverage the power of AI itself. Here are some approaches to consider:
- AI-Powered Threat Detection: Utilize AI and machine learning to enhance threat detection capabilities. AI can analyze vast amounts of data to identify patterns and anomalies that may indicate a security threat.
- Automated Incident Response: Implement AI-driven automation to streamline incident response processes. This can help organizations respond to threats more quickly and efficiently.
- Secure AI Development: Ensure that AI systems are developed with security in mind from the outset. This includes implementing secure coding practices, conducting regular security assessments, and using robust encryption methods.
- Ethical AI Practices: Adopt ethical AI practices to address privacy and bias concerns. This involves implementing transparency, accountability, and fairness in AI systems.
The traditional approach to data security, characterized by discovery, classification, rule definition, and enforcement, has proven to be insufficient in the face of modern cyber threats and the complexities introduced by AI. Continuing to rely on these outdated methods is, indeed, a form of insanity. To protect their data effectively, organizations must embrace a paradigm shift towards adaptive, proactive, and AI-enhanced security strategies. By doing so, they can break free from the cycle of repeated failures and build a more resilient defense against the ever-evolving landscape of cyber threats.